From idea to production
Expertise for your product - in all phases
ALL YOU NEED. That’s our promise to our customers – and it includes far more than high-tech mechanical engineering. More than production and packaging systems across all industrialization stages and comprehensive services. We design and realize highly specialized process solutions for pharmaceutical and medical technology companies worldwide, as well as for consumer and home care products from renowned brands. We contribute our expertise and experience throughout the product lifecycle and are your development partner for innovations right from the initial idea. And we shape a process that grows from the lab scale to fully automated high-performance production.
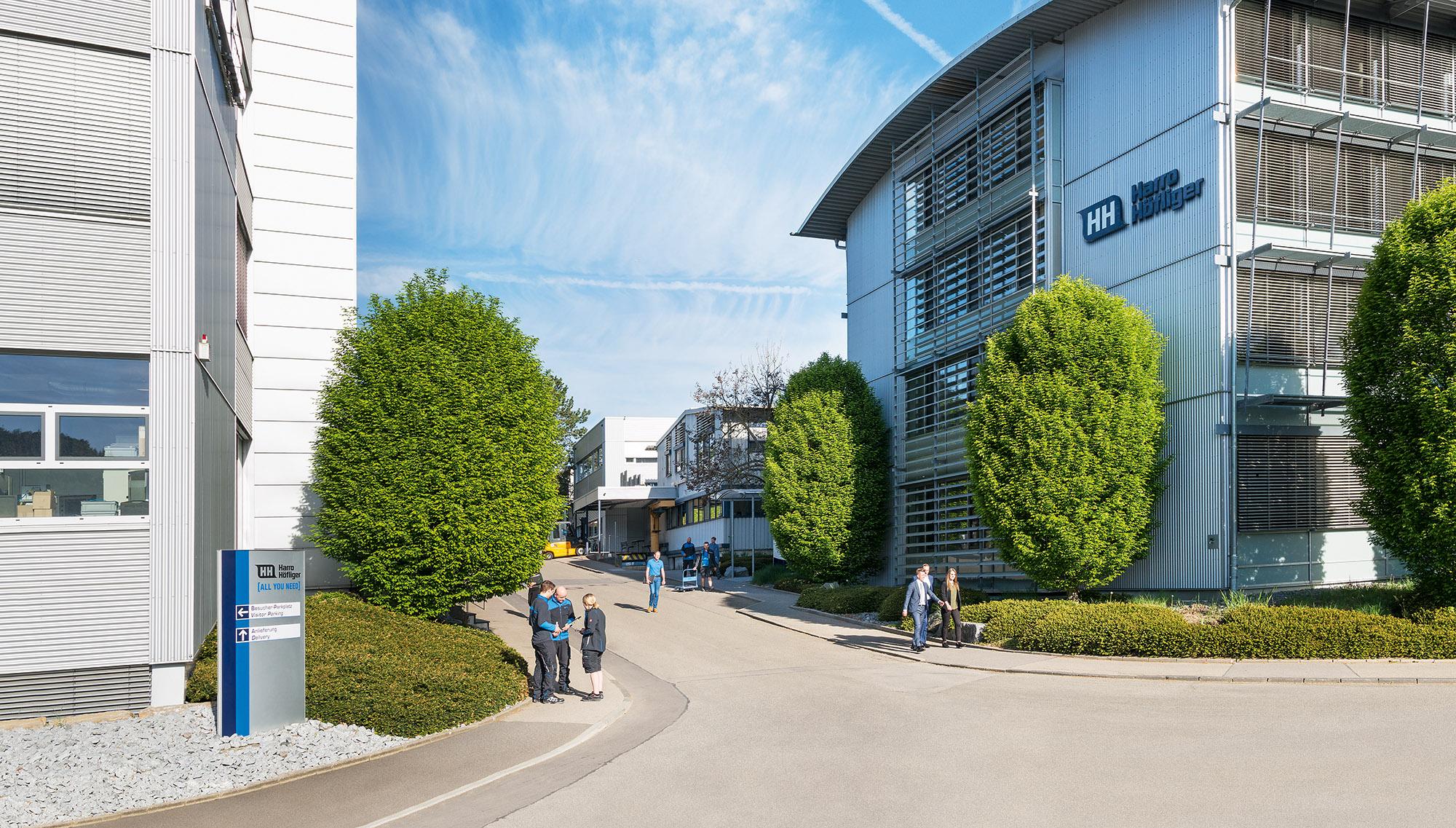
Who is Harro Höfliger?
More about usFor the success of your product
Complete expertise
We contribute our expertise across all areas to your projects – developing new solutions for innovative products and pharmaceutical forms. We offer sophisticated machine platforms for every stage of development and a broad foundation of key processes developed in-house – creating large-scale solutions like turnkey lines and set standards on a smaller scale in delicate assembly processes, for example. And with our services, we are at your side as a reliable partner all the way.
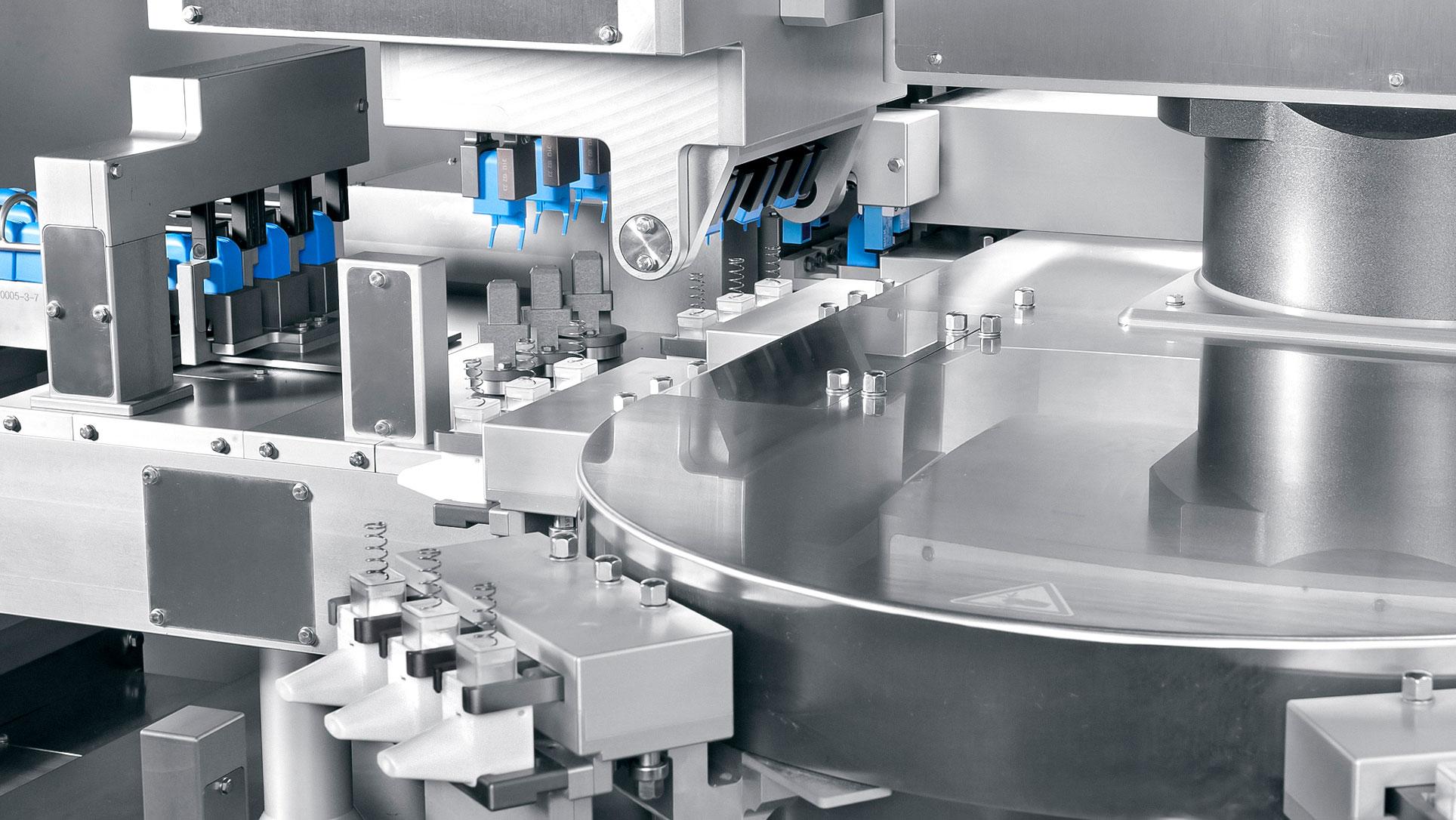
Technologies and know-how
Complex assembly tasks, dosing in the micro or macro range, web processing of diverse materials: Just a few of the tasks for which we offer in-house developed, field-proven technologies.
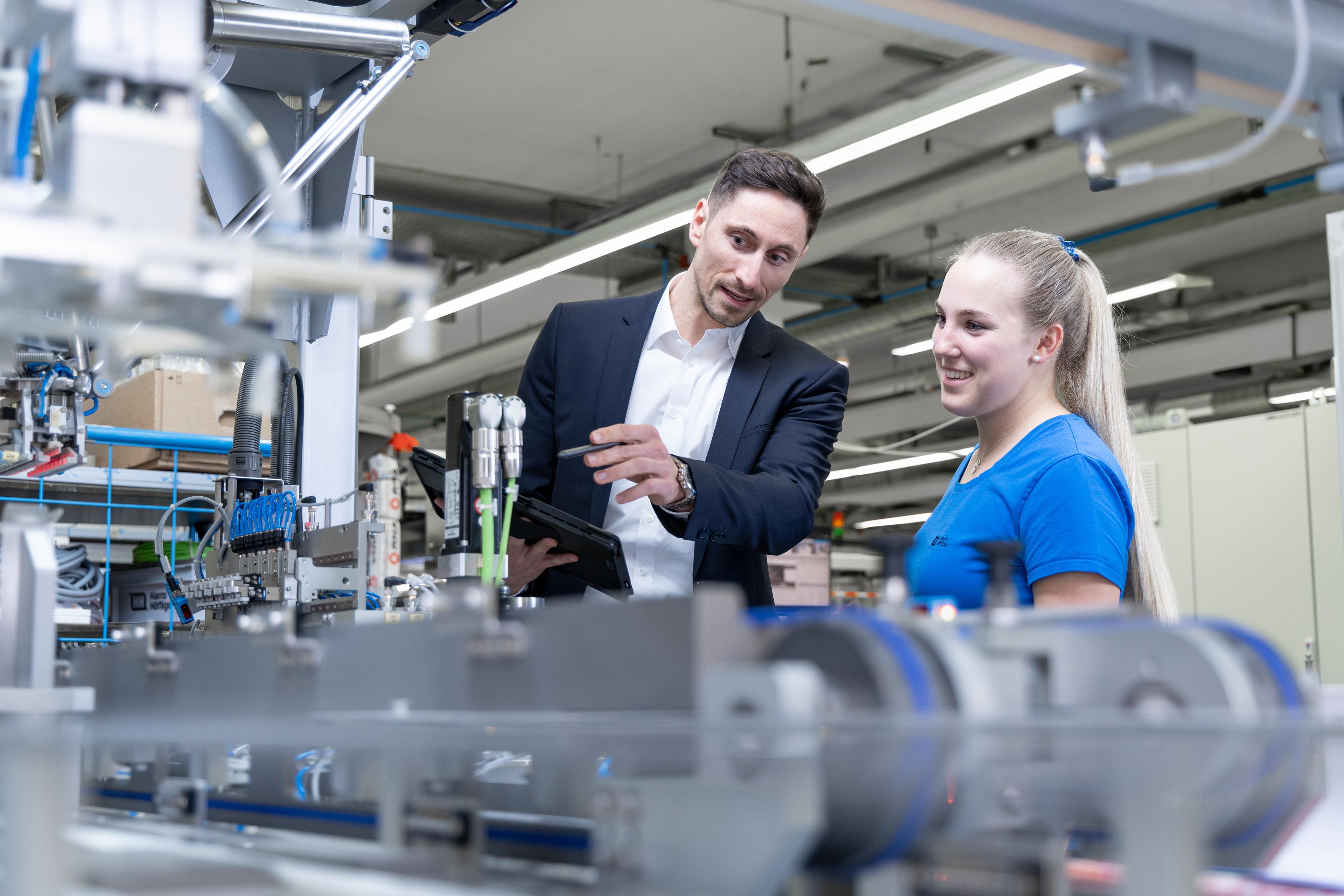
Trade shows and events
Upcoming events
PHI SEA 2025
Kuala Lumpur, Malaysia
Medical Technology 2025
Galway, Ireland
ISPE Singapore 2025
Singapore, Singapore
Farma Forum 2025
Madrid, Spain